Introduction
Imagine a world where companies can collaborate to train AI models, sharing insights and improving security, without ever risking the exposure of sensitive data. Sounds like a cybersecurity dream, right? Welcome to Federated Learning—AI’s collaborative superpower. In this post, we’ll dive into how this innovative technique allows organizations to build stronger, more secure AI models while ensuring data privacy. Whether you're a CTO, CISO, or compliance head, Federated Learning is something you need to know to stay ahead of the curve.
Abstract
Overview
Federated Learning is like the AI version of working together without actually sharing the work. Instead of sending sensitive data to a central server, each participant trains a model locally on their own data. The results are then aggregated and sent to the central server, but no raw data is exchanged. This method is a game-changer for privacy-preserving AI systems and has enormous potential in enhancing cybersecurity by using diverse data sources to create more accurate and robust models. It’s AI that’s smarter, safer, and more collaborative.
In the world of cybersecurity, the challenge of securing sensitive data while still harnessing the power of Artificial Intelligence (AI) is monumental. Enter Federated Learning—an AI technique that enables multiple organizations to collaboratively train machine learning models without ever sharing sensitive data. This not only enhances privacy but also strengthens cybersecurity systems by learning from diverse sources. In this blog, we explore how Federated Learning is redefining the way AI systems can improve security, while keeping your data private and protected.
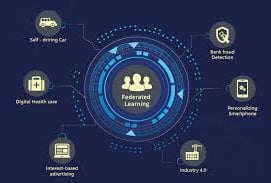
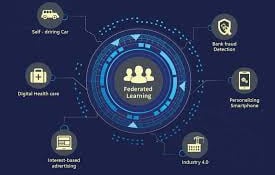
[Disclaimer: This blog post is for informational purposes only and should not be construed as legal or financial advice. Organizations should consult with legal counsel and regulatory authorities to ensure compliance with reporting requirements.]
Mandatory
For professionals in cybersecurity and compliance, keeping up with Federated Learning is no longer optional. The growing emphasis on data privacy regulations, like GDPR and CCPA, means that understanding how to use AI without compromising privacy is crucial. Federated Learning helps businesses comply with these regulations while still allowing them to utilize cutting-edge AI technology. This approach doesn’t just promise stronger security—it’s a necessity for staying compliant in today’s data-driven world.
Applicability
Federated Learning is applicable across many sectors, from healthcare and finance to government and beyond. If your organization handles sensitive data and needs to leverage AI for threat detection or fraud prevention, Federated Learning can help. It allows you to develop stronger, privacy-respecting models without the need to compromise on the quality or effectiveness of your AI-driven security systems. Think about a bank training an AI model for fraud detection using customer data—without ever sharing that data with anyone else.
Regulatory or Company Interest?
As data privacy concerns rise and regulations become more stringent, Federated Learning offers a vital solution. It’s a tool that helps organizations balance the need for robust cybersecurity measures with the demand for privacy. GDPR, HIPAA, and other data protection laws make it difficult to share sensitive data across borders or between companies. Federated Learning allows organizations to comply with these regulations while still benefiting from AI’s power, creating a new standard for privacy-preserving, collaborative learning in cybersecurity.
Key Guidelines:
Privacy by Design: Federated Learning’s core principle is that privacy is integrated into the process. Data remains on-site, and only model updates are shared, ensuring that sensitive information stays private.
Decentralized Learning: Participants train their models locally, reducing the need to centralize sensitive data while still benefiting from collective intelligence.
Collaboration without Data Sharing: This method allows different organizations to collaborate on training AI models, sharing knowledge without exposing private data.
Security Enhancement: By aggregating data insights across different sources, Federated Learning enables more accurate and robust AI models that improve threat detection, anomaly spotting, and fraud prevention.
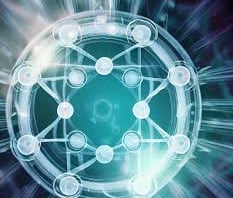
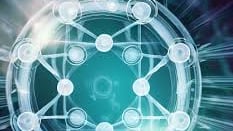
Key Implications
The implications of Federated Learning for cybersecurity are profound. By enabling multiple organizations to train models together without sharing data, it reduces the risks of data breaches and unauthorized access. This leads to:
Improved Threat Detection: With a broader base of data, AI models can recognize threats more accurately and in real-time.
Stronger Privacy: Organizations can comply with privacy laws while still benefiting from AI’s power.
Enhanced AI Models: Federated Learning helps develop AI systems that are better at spotting complex threats across industries without compromising privacy.
Countries with Adoption or Influence
Federated Learning is gaining traction globally, particularly in countries with stringent data privacy laws like the European Union (GDPR), Canada, and Japan. These regions are seeing an increased adoption of privacy-preserving technologies like Federated Learning to meet the needs of privacy-conscious users and regulatory bodies. Countries such as the U.S. are also exploring Federated Learning, especially in sectors like healthcare, finance, and government, where both data security and privacy are paramount.
International Frameworks Influenced
Federated Learning is having a significant influence on international data privacy frameworks. Standards like the General Data Protection Regulation (GDPR) and Health Insurance Portability and Accountability Act (HIPAA) now see Federated Learning as a potential solution to meet privacy requirements while using AI. As the technique continues to grow in popularity, we can expect to see more global frameworks incorporating it as a best practice for privacy-preserving AI models.
Regional and Industry-Specific Frameworks
Federated Learning is already impacting regional and industry-specific frameworks:
Healthcare: Healthcare organizations are adopting Federated Learning to train models on patient data across different institutions while maintaining HIPAA compliance.
Financial Services: In the finance industry, Federated Learning allows financial institutions to collaborate on fraud detection models without violating confidentiality agreements or sharing sensitive customer data.
Government and Defense: Federated Learning is also being explored for use in government sectors to enhance national security models by pooling data from various agencies without compromising privacy.
Secure Your Digital Identity with SecureKnots
Contact us to learn more about our cybersecurity services and ensure your organization meets cybersecurity requirements.


Conclusion
Federated Learning is revolutionizing the world of cybersecurity by providing a way for multiple organizations to collaborate, learn from diverse datasets, and build stronger, more accurate AI models—all without compromising data privacy. Whether you’re a CTO, CISO, or compliance head, understanding and implementing Federated Learning in your organization can boost your cybersecurity posture while ensuring compliance with global data protection regulations.
By exploring the power of Federated Learning, we've outlined how this technology will shape the future of cybersecurity. But this is just the beginning. Stay tuned to our blog for more insights on emerging technologies and strategies to stay one step ahead of cyber threats.
Thank you for your attention! If you have any inquiries about cybersecurity requirements or need expert guidance, please don't hesitate to contact SecureKnots.
This should wrap up the blog and fulfill the promise made in the previous one! Feel free to adapt or modify any section to suit your tone and objectives better.
Federated Learning: AI’s Collaborative Superpower in Cybersecurity